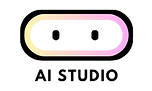
Create Your First Project
Start adding your projects to your portfolio. Click on "Manage Projects" to get started
Spam Email Classifier
Tipo de projeto
AI Model
Data
2023
Local
Amsterdam
When it comes to building a spam email classifier, it's crucial to evaluate its performance. Let's dive into the key metrics used to assess the effectiveness of such a classifier.
1. Accuracy: 📊
Accuracy measures how well our classifier predicts whether an email is spam or not. In your results, you achieved an impressive accuracy of approximately 99.19%. This means that the classifier correctly identified over 99% of the emails in the test dataset. A high accuracy indicates the model's overall effectiveness.
2. Confusion Matrix: 📉
The confusion matrix breaks down the classifier's performance in more detail:
True Positives (TP): 140 spam emails correctly identified as spam.
False Positives (FP): 0 ham (legitimate) emails incorrectly classified as spam.
True Negatives (TN): 966 ham emails correctly identified as ham.
False Negatives (FN): 9 spam emails incorrectly classified as ham.
3. Classification Report: 📈
The classification report provides a summary of essential metrics:
Precision: Measures the proportion of correctly predicted spam emails among all emails predicted as spam. In your case, it's 1.00, indicating that when the model predicts an email as spam, it's almost always correct.
Recall (or Sensitivity): Measures the proportion of correctly predicted spam emails among all actual spam emails. An impressive 0.94 means your model accurately identifies 94% of actual spam emails.
F1-score: This is the harmonic mean of precision and recall, offering a balanced view of both. An F1-score of 0.97 for spam is commendable.
Support: Reflects the number of occurrences of each class in the test dataset.
In conclusion, your spam email classifier is performing exceptionally well, boasting a high accuracy and robust precision, recall, and F1-score for spam emails. The limited number of false negatives (9) highlights the model's ability to catch most spam emails effectively.
Understanding these metrics is vital for fine-tuning a spam email classifier and ensuring accurate email categorization, ultimately contributing to a cleaner and more secure inbox.